
However, few of them addressed the both steps.įundamental analysis is the most common way to assess firm value. iterature referred to this paper is classified as fundamental analysis and financial networks. The process of portfolio optimization is decomposed into steps as asset selection and capital allocation. The fundamental networks bridge portfolios to fundamental information with network theory. The capital allocated to a stock is proportionate to the concept of weighted degree in network theory. As a proxy for a portfolio, a fundamental network is defined as a set of “interconnected” stocks, among which linkages are a measure of similarity of fundamental information among stocks. Compare to it, FN avoids the estimation errors of expected return and standard deviation,and also removes the requirement of a positive definite co-variance matrix. It can be used as an alternative to the classical Markowitz’s model. In this paper, we propose a novel approach for portfolio optimization, referred to as “Fundamental Networks” (FN), an effective and robust network-based fundamental-incorporated method. Therefore, the main purpose of our research is to bridge portfolio optimization to fundamental analysis with network structure. However, few of them conveyed fundamental information. The application of financial networks started a new branch in optimizing portfolios. Studies introduced fundamental analysis to mean-variance model are still confined by the drawbacks. To our knowledge, substitution of mean-variance framework has not been well discussed in the area of portfolio optimization. Moreover, MPT only involves the analysis of prices and is not able to incorporate the fundamental analysis which has been deemed value-relevant to stock return by not only well-known investors mentioned earlier, but also multiple researchers in the past. Third, the lack of hierarchical structure in a correlation matrix allows weights to vary freely in unintended ways, which is a root cause to the mean-variance efficient portfolio’s instability. Hence, the portfolio cannot be constructed for a large number of stocks. Second, the mean-variance optimization requires the inversion of a positive-definite co-variance matrix which would lead to large estimation errors and will further offset the benefits of diversification. First, the high sensitivity of the estimated mean-variance efficient portfolio to estimation errors in expected return may lead to non-robust results. However, within the last few decades, practitioners and academics have become aware of its drawbacks.
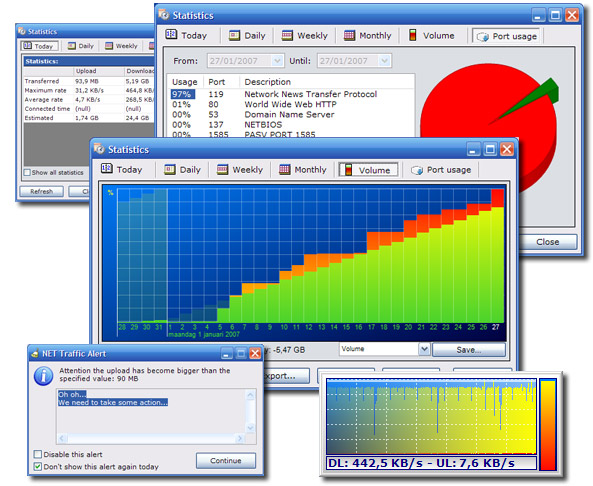
Modern Portfolio Theory (MPT) has served as the foundation and industry standard for portfolio optimization, being capable of demonstrating the concepts of diversification and risk-return efficiency based on a mean-variance framework. The problem of portfolio optimization is one of the most important issues in asset management.
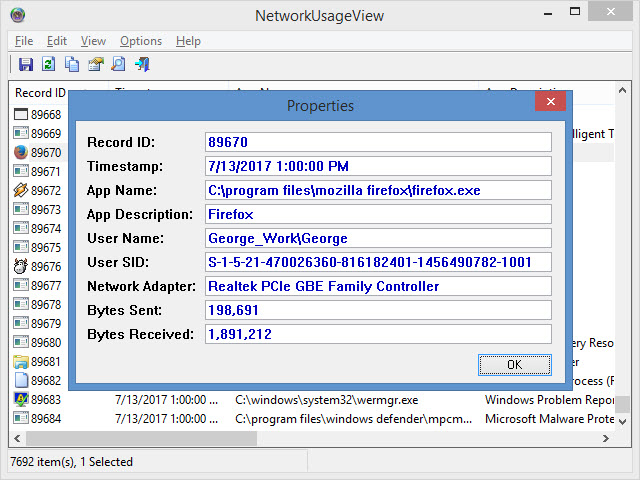
Specifically, portfolios set for profitability goals create excess return in a general/upward trending market portfolios targeted for operating fitness perform better in a downward trending market, and can be considered as a defensive strategy in the event of a crisis. We find that Fundamental Networks efficient portfolios are in general more mean-variance efficient in out-of-sample performance than Markwotiz’s efficient portfolios.

Two empirical models are provided in this paper as applications of Fundamental Networks. As a proxy for a portfolio, a fundamental network is defined as a set of “interconnected” stocks, among which linkages are a measure of similarity of fundamental information and are referred to asset allocation directly. FN is an effective and robust network-based fundamental-incorporated method, and can be served as an alternative to classical mean-variance framework models. This article proposes the use of a novel approach to portfolio optimization, referred to as “Fundamental Networks” (FN).
